Looking Into Your Speech: Learning Cross-Modal Affinity for Audio-Visual Speech Separation
Author | Jiyoung Lee, Soo-Whan Chung, Sunok Kim, Hong-Goo Kang, Kwanghoon Sohn |
Publication | IEEE/CVF Conference on Computer Vision and Pattern Recognition (CVPR) |
Month | June |
Year | 2021 |
Link | [Paper] [Project] |
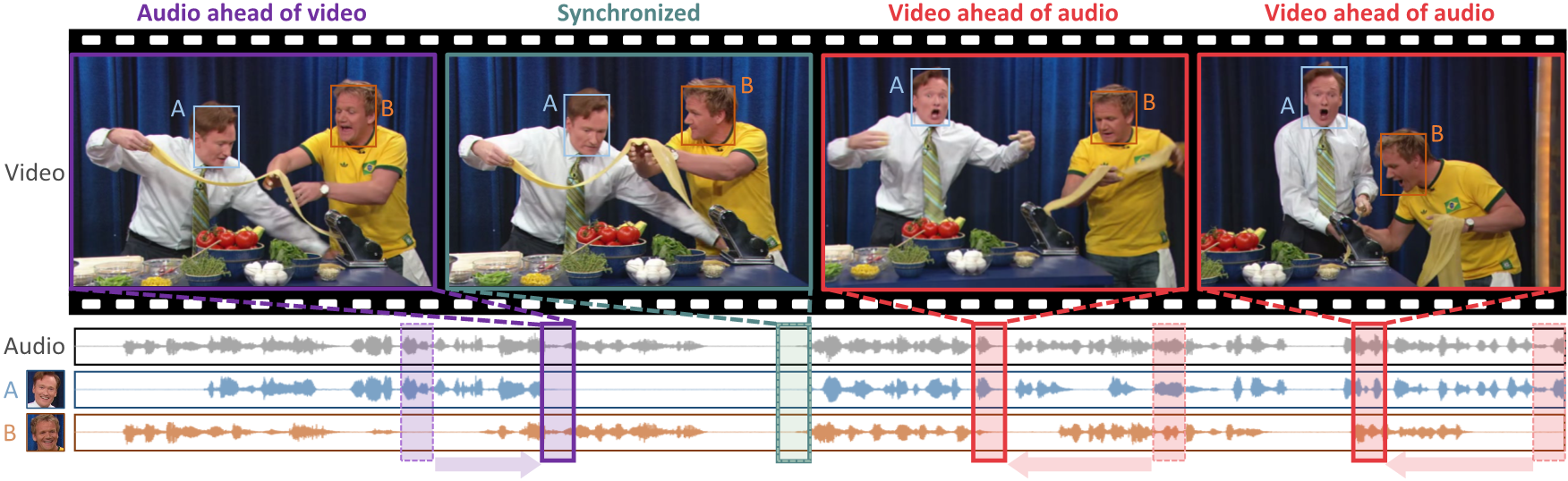
We wish to hear individual speech of a desired speaker only even if there is frame discontinuity in the audio-visual data. When audio and video segments are taken from different points in time (solid box), it is intuitively difficult to separate speech of each speaker compared to the aligned cases (dashed box).
ABSTRACT
In this paper, we address the problem of separating individual speech signals from videos using audio-visual neural processing. Most conventional approaches utilize frame-wise matching criteria to extract shared information between co-occurring audio and video. Thus, their performance heavily depends on the accuracy of audio-visual synchronization and the effectiveness of their representations. To overcome the frame discontinuity problem between two modalities due to transmission delay mismatch or jitter, we propose a cross-modal affinity network (CaffNet) that learns global correspondence as well as locally-varying affinities between audio and visual streams. Given that the global term provides stability over a temporal sequence at the utterance-level, this resolves the label permutation problem characterized by inconsistent assignments. By extending the proposed cross-modal affinity on the complex network, we further improve the separation performance in the complex spectral domain. Experimental results verify that the proposed methods outperform conventional ones on various datasets, demonstrating their advantages in real-world scenarios.